Terms such as demand forecasting, bullwhips or "AI-powered demand planning" are part of the vocabulary of the modern supply chain - in other words, supply chain management.
So let's first clarify what terms such as demand forecasting, demand planning, demand forecasting or demand planning mean. Demand forecasting or demand forecast is the English word for demand forecast. Demand planning is the translation for demand planning. Let's take a closer look.
Demand Forecasting
Demand forecasting is the process of estimating future demand by analysing data. Put simply, it means predicting how much consumers will be interested in buying a product. These predictions are based on historical sales data, current market trends and other external factors.
Demand Planning
Demand planning is an overarching process that not only uses predictive demand forecasting, but also includes the planning and management of inventory levels, production and procurement strategies. It is about ensuring that the right product is available in the right place at the right time to fulfil the predicted demand. In a separate article, we will take a closer look at the different requirements for the two concepts mentioned.
This article deals with the question of how the application of artificial intelligence affects demand forecasting. It is also called "Predictive Analytics for Demand Forecasting".
You might think that supply chains, as prime examples of demand forecasting and general demand planning, have long been professionalised from top to bottom. Not at all.
Status Quo Supply Chain Management
A 2021 McKinsey report found that nearly 75% of supply chain leaders rely on manual methods for supply chain planning, primarily spreadsheets. In other words, Excel. It's hard to believe. This reliance naturally increases the risk of human error and leads to time-consuming data collection and preparation, which often results in outdated information. In addition, companies usually work in isolated environments, which complicates the process, as technical systems such as artificial intelligence, among others, have to collect data from various independent sources.
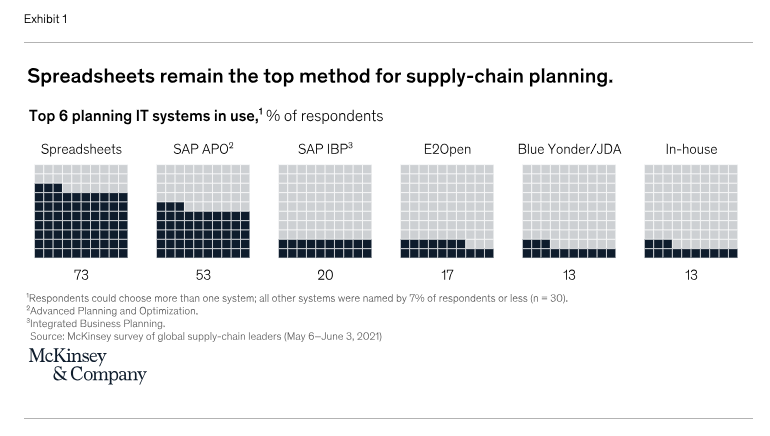
(Fig. 1: spreadsheets remain the top method for supply chain planning.
Simply networking data by breaking down data silos will provide an enormous performance boost. In combination with artificial intelligence, the industry is literally reaching the next level, which, according to the report "Global AI in Supply Chain Management (SCM) 2023-2028" by Research and Markets, looks as follows:
Artificial Intelligence (AI) in SCM will reach US$17.5 billion globally by 2028, with AI-powered supply chains being over 67% more effective thanks to reduced risk and lower overall costs.
That's good news. So now let's look at how artificial intelligence is reducing both risks and costs to make supply chain management more effective.
The main advantages of AI for demand forecasting
The integration of artificial intelligence (AI) into demand forecasting is unsurprisingly a major advance in the field of supply chain management. AI enables highly accurate forecasting of future demand, ensuring that it overcomes the limitations of traditional forecasting methods, which often rely heavily on past patterns and simplified assumptions. By using machine learning, AI algorithms are able to analyse vast amounts of data, both in the past and in real time, to identify complex patterns and trends that human analysis could easily overlook. This leads to more accurate and detailed forecasts, which are crucial for various industries such as retail, life sciences, the semiconductor industry or fintech (financial technology).
Let's take a look at this in more detail:
Efficiency in Data Handling
AI excels in structuring and analysing extensive datasets quickly and accurately. Unlike traditional methods that require extensive manual input, AI streamlines the data preparation process. This results in a more efficient demand planning process, freeing up time for strategic decision-making and reducing the risk of human errors. For example, according to Lloyd's Register (Maritime Performance Services), only 10 percent of ship data can be taken into account in shipping due to the large amount of data, whereas AI models can now view and immediately process almost 100 percent of ship data.
Precise and Dynamic Forecasts
AI offers a sophisticated approach to forecasting by autonomously selecting and combining appropriate methods for different products and levels. This granular approach leads to increased accuracy in forecasts, crucial for making informed decisions in the supply chain.
Comprehensive Data Integration
AI's ability to incorporate both internal and external data into forecasts sets it apart. It can identify causal relationships between various factors, such as market trends and economic data, that influence demand. This level of integration provides a more holistic view of the market, which traditional methods may overlook.
Rapid Responsiveness
AI rapidly adapts to market fluctuations, a critical advantage in today’s volatile environment. This adaptability is particularly valuable for responding to unexpected events or 'Black Swan' incidents, like the COVID-19 pandemic, which significantly affect demand patterns.
Enhanced Decision Making through Clarity
AI's role in demand planning marks a significant leap towards clarity in decision-making. By accurately detecting outliers, AI not only flags potential fluctuations but also provides a comprehensive understanding of their impact on the business. This early identification enables timely and effective interventions, ensuring that decisions are not just reactive but strategically aligned.
Improved Supply Chain Relationships
AI's accurate forecasting minimises the bullwhip effect and leads to better inventory levels. This, in turn, results in more efficient planning by supply chain partners, reducing stress and enhancing collaborative relationships.
Democratisation of Data Science
AI enables those without extensive data science expertise to perform complex demand forecasting. Its self-learning systems create and adapt models as needed, allowing team members to focus on strategic tasks while leaving the quantitative heavy lifting to AI.
Forecast Improvement Over Time
AI in demand forecasting continuously evolves and improves with each iteration of data analysis. Utilising machine learning, AI systems are capable of learning from new data, refining their forecasting models over time. This ongoing improvement leads to progressively more accurate predictions. As the system encounters more scenarios and integrates feedback, it becomes adept at recognizing subtle market trends and demand shifts. This dynamic learning process ensures that businesses are not only equipped with the current best forecasting models but are also positioned to adapt and evolve with changing market dynamics, maintaining a competitive edge in demand planning.
Overall, the trend is striking - these benefits show how artificial intelligence is transforming demand forecasting from a labour-intensive, error-prone process into a strategic, data-driven approach that improves efficiency, accuracy and responsiveness in supply chain management.
The challenges of AI for Demand Forecasting
There is no light without shadow. Although AI offers decisive advantages in predicting market trends and optimising inventory management, its use is not without its hurdles. These challenges range from technical and data-related issues to broader organisational and market-related factors. These challenges need to be understood and overcome in order to realise the full potential of AI in improving demand forecasting. Below we take a closer look at some of the key obstacles that organisations may face:
Data Quality and Integrity
AI models require high-quality, comprehensive data. Challenges arise when data is inconsistent, incomplete, or biassed, leading to inaccurate forecasts. Just like always, the well-known IT mantra applies: "garbage in, garbage out".
Integration with Existing Systems
Integrating technology into existing business processes and systems is often associated with technical challenges. This integration sometimes requires overcoming cultural resistance and ensuring compatibility with legacy systems.
Complexity of AI Models
Developing, interpreting, and managing AI models demand specialised knowledge. This complexity necessitates investment in skilled personnel or collaborations with AI solution providers.
Understanding AI Decisions
The 'black box' nature of some AI models can create transparency issues, making it difficult for managers to understand and trust the AI's decision-making process. Furthermore, misjudged AI decisions can cause all kinds of damage.
Global Uncertainties
Unforeseen events such as pandemics or economic fluctuations can jeopardise the predictive accuracy of AI. AI models need to be retrained or adapted quickly to adapt to new market conditions.
Continuous Model Management
Also, regardless of unpredictability, the dynamic nature of markets requires constant monitoring and updating of AI models to maintain their relevance and accuracy, which can be resource intensive.
To summarise, AI brings many transformative benefits for demand forecasting. However, its successful implementation requires overcoming challenges in terms of data quality, system integration and ongoing management. Companies can utilise the capabilities of artificial intelligence to improve their supply chain management in the areas of cost, risk and efficiency.
FAQs
What is AI-driven demand forecasting?
AI-driven demand forecasting is a method of using artificial intelligence and machine learning to predict future demand for products or services. This method is more accurate than traditional methods, which rely on historical sales data and simple statistical models.
How does AI help with demand forecasting for new products?
It uses existing product data to model demand until sales history is available.
How does AI improve traditional demand forecasting methods?
AI speeds up data processing, uncovers hidden problems, and creates more granular forecasts than traditional methods.
Can AI help in workforce planning and customer satisfaction?
Yes, AI aids in forecasting staff demand and ensures product availability, leading to higher customer satisfaction.
What are some types of AI demand forecasting?
AI demand forecasting includes short-term, long-term, active, passive, internal, and external types, each suitable for different business needs.
What are the limitations of traditional demand forecasting methods?
Traditional methods often rely on historical data and assume past patterns will continue. They may struggle to incorporate external factors like market trends and unexpected events, leading to inaccurate forecasts.
How does AI improve demand forecasting?
AI analyses vast amounts of data, including external factors, to identify complex patterns. This leads to more accurate and comprehensive forecasts, optimising inventory management and reducing waste.
Can AI-driven demand forecasting adapt to market changes and uncertainties?
Yes, AI can be particularly effective in adapting to rapid market changes and global uncertainties such as economic downturns or pandemics. It analyses real-time data to adjust forecasts accordingly. However, errors can also occur here.
What are the overall benefits of AI in demand forecasting for supply chain management?
AI-driven demand forecasting enhances supply chain efficiency and profitability. It helps businesses overcome traditional forecasting limitations, navigate complexities, and adapt to changing market conditions.