Processing large amounts of data is one of the favourite problems of artificial intelligence – thank God! By 2025, an annual 175 zettabytes (1 zettabyte = 1 trillion gigabytes or 1 billion terabytes) of data is currently expected (see Fig. 1). Huge amounts of data are also being generated in the course of sustainability initiatives. AI supports us in meeting this challenge – in particular in analysing, processing and using this data to ultimately make informed decisions.
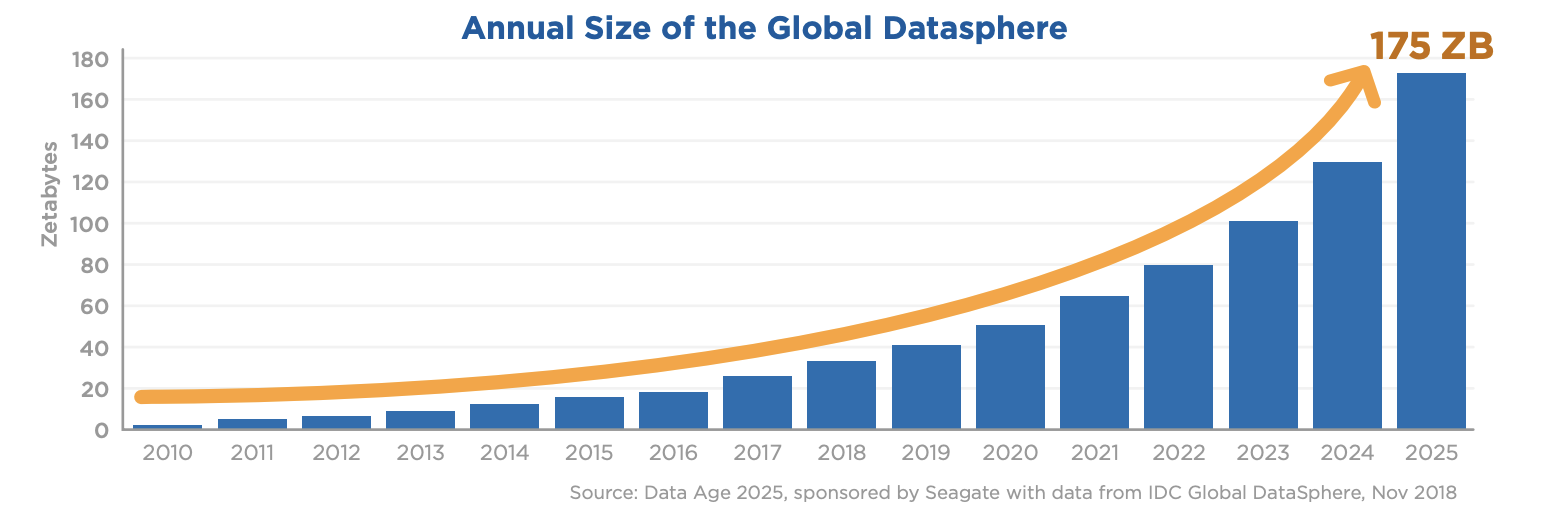
Challenges arising from the flood of data in the sustainability sector
We will start by looking at the challenges that large amounts of data bring with them. In particular, these challenges relate to the growth, complexity, quality and security of data.
Exponential growth rates in data volume
Regulatory frameworks such as the Corporate Sustainability Reporting Directive (CSRD) and other sustainability efforts are requiring companies to collect more and more data. This includes information on CO2 emissions, energy consumption, supply chains, resource consumption and much more. The amount of data is growing exponentially (see Fig. 1) and is therefore naturally becoming confusing. The challenge is to structure and store this data efficiently in order to make it available for sustainability analysis and reporting.
Complexity and heterogeneity of the data
In addition to the sheer volume of data, its heterogeneity is another challenge. This is a general big data problem. Sustainability data comes from a variety of sources, such as internal systems, external databases, sensors, supplier information and public reports. This data is available in different formats, structures and qualities, which makes it difficult to integrate and analyse. There is often a lack of standardised formats and interfaces that enable seamless data merging. The result? Inconsistencies and data gaps that affect the quality and validity of sustainability analyses.
Data quality and reliability
The next critical aspect is ensuring data quality. Organisations must make sure that the data collected is, to put it simply, accurate, complete and up to date. We have written an entire article on this topic: ‘What is data quality and what must companies carefully comply with?’
Incorrect or inaccurate data leads to incorrect analyses and thus to poor decisions that jeopardise sustainability goals. Such mistakes can also land companies in hot water legally. The trustworthiness of data sources follows directly as the next issue. Here, too, companies must ensure that they identify and use reliable and valid data sources.
Security and data protection concerns
The collection and processing of large amounts of sustainability data also entails particular security and, to some extent, data protection risks. Cyberattacks have been on the rise for some time and are a global problem. Appropriate measures must be taken to protect data against unauthorised access, misuse and cyberattacks. Data protection laws, such as the GDPR, set high standards for the handling of personal data – however, the amount of this data is rather small when it comes to sustainability. Overall, the challenge is to strike a balance between data security and openness to innovation and collaboration.
The role of artificial intelligence in data processing
AI to the rescue! Big challenges need big ideas and innovative technologies. AI is this key technology. Now we are highlighting how AI effectively manages complex and diverse data streams in the area of sustainability and thus supports decision-making for companies.
Automation and increased efficiency through AI
One of the key capabilities of AI is the automation of data processing. Manual data collection and processing is a thing of the past – AI-based systems process and analyse large amounts of data in real time. This results in a significant increase in efficiency. In particular, machine learning algorithms are able to recognise patterns and anomalies in the data that would be almost impossible for a human analyst to detect. This enables companies to react more quickly to changes and proactively take measures with regard to sustainability strategies.
Improving data quality and consistency
As discussed in the first section, data heterogeneity is both important and challenging. AI can harmonise data from different sources and make it consistent. Through advanced data mining techniques and natural language processing (NLP), AI can clean data, delete duplicates and ensure that the remaining data is accurate and consistent. This improved data quality lays the foundation for reliable analysis and reporting, which are fundamental to achieving sustainability goals.
Predictive capabilities and proactive sustainability strategies
A particular advantage of AI is its ability to predict. Machine learning algorithms forecast future trends and patterns, enabling organisations to proactively plan and implement sustainable measures. For example, AI can be used to predict a company's energy consumption or emissions and make appropriate suggestions for optimisation. These proactive approaches not only help companies achieve their own sustainability goals, but also meet regulatory requirements and strengthen their reputation as a sustainable business.
Decision-making assistance
AI also enables companies to make more informed decisions. This is possible thanks to comprehensive analyses and deep insights into the data. This is particularly important in the context of sustainability, where decisions are complex and often fraught with uncertainty. AI-based systems help decision-makers to better understand risks and opportunities and develop evidence-based strategies. In procurement and supply chain planning (next chapter), AI plays a central role in effectively implementing and holistically monitoring sustainability criteria.
Optimisation of resources and reduction of environmental impact
By applying AI, companies can optimise their resource allocation and thus further minimise their environmental impact. AI helps to monitor resource consumption more closely and identify inefficient processes. Classic examples of optimisation in supply chain demand management for lower CO2 emissions are demand forecasting and demand planning. This targeted resource utilisation not only contributes to sustainability, but also usually means significant cost savings for companies.
Improving decision-making in purchasing
Next, let's look at procurement. Purchasing plays an important role in implementing sustainability strategies because it is crucial for selecting suppliers, materials and products. However, with the increasing flood of data in sustainability, it is becoming more and more difficult for procurement to make informed decisions. Artificial intelligence offers full support here by optimising decision-making processes through data-driven analysis, thus enabling more sustainable procurement.
Analysing and evaluating suppliers
One of the biggest challenges in sustainable procurement is evaluating and selecting suppliers, which must meet the company's sustainability criteria. Traditional methods of supplier evaluation are often based on historical data and subjective assessments, which makes it difficult to capture current sustainability performance. This is where AI comes in, analysing large amounts of data in real time to provide a comprehensive picture of actual supplier performance. Machine learning algorithms can, for example, evaluate sustainability reports, certifications and publicly available data sources to provide an objective and up-to-date assessment. If ‘cheating’ occurs at any point, ML can detect such cases.
The application of AI also helps to identify potential risks in the supply chain at an early stage. Using historical data and predictive analytics, potential disruptions or compliance violations can be predicted, enabling a proactive risk management strategy. The result: a more reliable selection of suppliers that not only deliver high-quality products but also contribute to the company's sustainability goals.
Optimisation of material
Material selection is another critical aspect of purchasing decisions. With the help of AI, companies can better evaluate the sustainability of materials and consider alternative options that are more environmentally friendly and cost-effective. AI-powered systems analyse the environmental impact of different materials based on factors such as CO2 emissions, energy consumption and recyclability.
This enables procurement to make decisions that take into account both economic and ecological criteria. And it doesn't stop there. In addition, AI can
make suggestions for innovative, more sustainable materials that may not have been considered before, thus helping to promote sustainable innovation.
Cost-benefit analysis
Conducting a data-driven cost-benefit analysis is essential to evaluate the economic impact of sustainable purchasing decisions. AI systems can process large amounts of data to calculate the total cost of sustainable procurement strategies,including hidden costs such as energy consumption and waste management. By using AI to analyse data from multiple sources, including ERP systems and external databases, companies can perform an accurate cost analysis and demonstrate the long-term financial benefits of sustainable decisions.
It is also possible to simulate different scenarios, modelling different purchasing strategies and their potential impact on sustainability and the bottom line. This helps companies find the optimal balance between cost, quality and sustainability.
Personalised purchasing decisions
By integrating AI into purchasing systems, individual sustainability requirements and preferences can be automatically incorporated into the decision-making process. For example, AI can analyse historical purchasing data and current market trends to provide tailored recommendations for more sustainable products or services. This results in a better alignment of the purchasing strategy with the company's overarching sustainability goals.
Support for compliance and transparency
Organisations are currently placing an ever-greater emphasis on compliance. This is because adhering to sustainability standards and regulations is becoming increasingly complex. Thus, a corporate risk. AI can help procurement minimise compliance risks by continuously monitoring data from various sources and checking for violations of established sustainability guidelines. AI-based systems also improve traceability and transparency in the supply chain. Data from various stages of the supply chain is continuously integrated and analysed to keep potential compliance risk low.
Supply chain visibility is a topic in itself, which is why we are now delving deeper into the topic of supply chain transparency and exploring how AI can help optimise the entire supply chain.
Optimisation of supply chain visibility
In a globalised world, supply chains are more complex and opaque than ever before. The challenge of tracking sustainability standards is a clear one – even for artificial intelligence. However, here too, AI offers solutions that significantly improve transparency in supply chains.
The importance of transparency and sustainability
A transparent supply chain enables companies to track the origin and path of their products – from raw material to end consumer. This is the only way to ensure thatsustainability criteria are met and that ethical and ecological standards are respected throughout the entire supply chain. Transparency builds consumer trust and minimises risks such as environmental violations or negative social impacts. The challenge here, too, lies in the tremendous amount of data that is collected along the entire supply chain.
Data collection and analysis with AI
As mentioned earlier, AI collects, integrates and analyses large amounts of data from various sources to obtain a holistic view of the supply chain. Sensors and IoT devices collect data in real time about the condition and movement of products and are one of the reasons for the high volumes of data. This data feeds into AI systems that can recognise patterns and identify deviations/anomalies that indicate problems or risks.
For example, AI can monitor emissions and energy consumption along the supply chain and help ensure that sustainability targets are met. Continuous data analysis enables seamless monitoring of the supply chain, significantly increasing transparency.
Prediction and risk management
Another advantage of AI in supply chain visibility is its forecasting and risk management capability. By analysing historical data, ML can identify potential risks and bottlenecks in the supply chain at an early stage. This enables companies to take proactive measures to prevent or mitigate disruptions.
These predictive capabilities enable companies to increase the resilience of their supply chains while ensuring that sustainable practices are adhered to. Organisations can not only respond to short-term disruptions, but also develop long-term strategies to improve sustainability.
Improve communication and collaboration
Transparency also requires effective communication and collaboration between the various supply chain stakeholders. AI-based platforms can serve as centralised hubs where information is shared in real time. This fosters collaboration between suppliers, manufacturers and retailers, creating a shared understanding of sustainability goals and practices.
AI also makes it possible to share information with relevant stakeholders by automating communication and highlighting important data and insights. Through this improved communication, companies can ensure that all parties are aware of sustainability standards and understand their roles and responsibilities in the supply chain.
Blockchain technology and AI
An area of interest in supply chain transparency is the combination of AI and blockchain technology. While blockchain provides the immutable record and secure storage of transactional data, AI can provide the analysis and interpretation of that data. Together, these technologies provide a robust solution for ensuring supply chain transparency. The blockchain guarantees the immutability of the data, while AI provides an in-depth analysis to identify any violations of sustainability guidelines.
Integration of sustainability data into existing procurement processes
Procurement processes often take years to mature. However, sustainability data can be integrated into these processes retrospectively in order to anchor sustainable business practices directly in the corporate strategy. This integration can be more or less challenging – because it is not only about collecting and analysing data – but also about organisational and technical implementation. That is why we will conclude by looking at how organisations successfully integrate sustainability data into their procurement processes and what advantages they derive from doing so.
Processes for integrating sustainability data
Integrating sustainability data into the procurement process requires a systematic approach. Organisations must first define which sustainability criteria are relevant to them, based on their strategic goals and regulatory requirements. These include, among others, environmental standards, ethical labour practices and resource conservation.
Next, it is important to integrate these criteria into supplier evaluation and selection. Companies should regularly monitor their suppliers for compliance with these criteria and conduct audits to ensure that the defined standards are met. AI can also be used here. For example, AI-supported audit systems that enable continuous monitoring and reporting.
A further step is to automate procurement decisions based on this sustainability data. This can be done by integrating sustainability metrics into existing enterprise resource planning (ERP) systems, so that purchasers can automatically choose the most sustainable options in their daily decisions.
Training & Awareness
In addition to technological adaptation, change management is the most challenging step – because it involves a change in corporate culture. And that means a change in behaviour. Therefore, all parties involved – especially procurement – must be sensitised and trained in the topic of sustainability. When employees develop a deep understanding of why sustainability is important and how they can contribute to it through their decisions, it is easier to change their behaviour.
Training programmes and workshops are particularly helpful in raising awareness and understanding the added value of sustainability data in procurement. Clear communication channels are also immensely helpful for sharing the successes of sustainable procurement strategies and thus strengthening employee engagement.
Benefits of integration
Integrating sustainability data into existing purchasing processes offers numerous advantages, some of which we have already mentioned. Companies can demonstrate their environmental and social responsibility and thus gain the trust of customers, investors (especially with regard to ESG) and other stakeholders. Furthermore, the use of sustainability data leads to a better risk management strategy, as possible violations of sustainability standards can be identified and remedied at an early stage.
In the long term, sustainable procurement leads to cost savings through more efficient processes and better waste management. A company's innovative strength can also be increased by focusing on sustainability, as it constantly seeks new, more environmentally friendly and efficient solutions.
Integrating sustainability data into existing purchasing processes is not a one-time effort, but an ongoing process that requires technological, organisational and cultural adjustments. Classic change management, in other words. With the right strategy and the right technologies, in particular the use of AI, companies can not only achieve their sustainability goals but also increase their competitiveness. In this article, we have presented the basics of this integration. Future developments will show how companies can further refine and apply these concepts to create a more sustainable future.